Enhancing Business Efficiency through Machine Learning Data Annotation
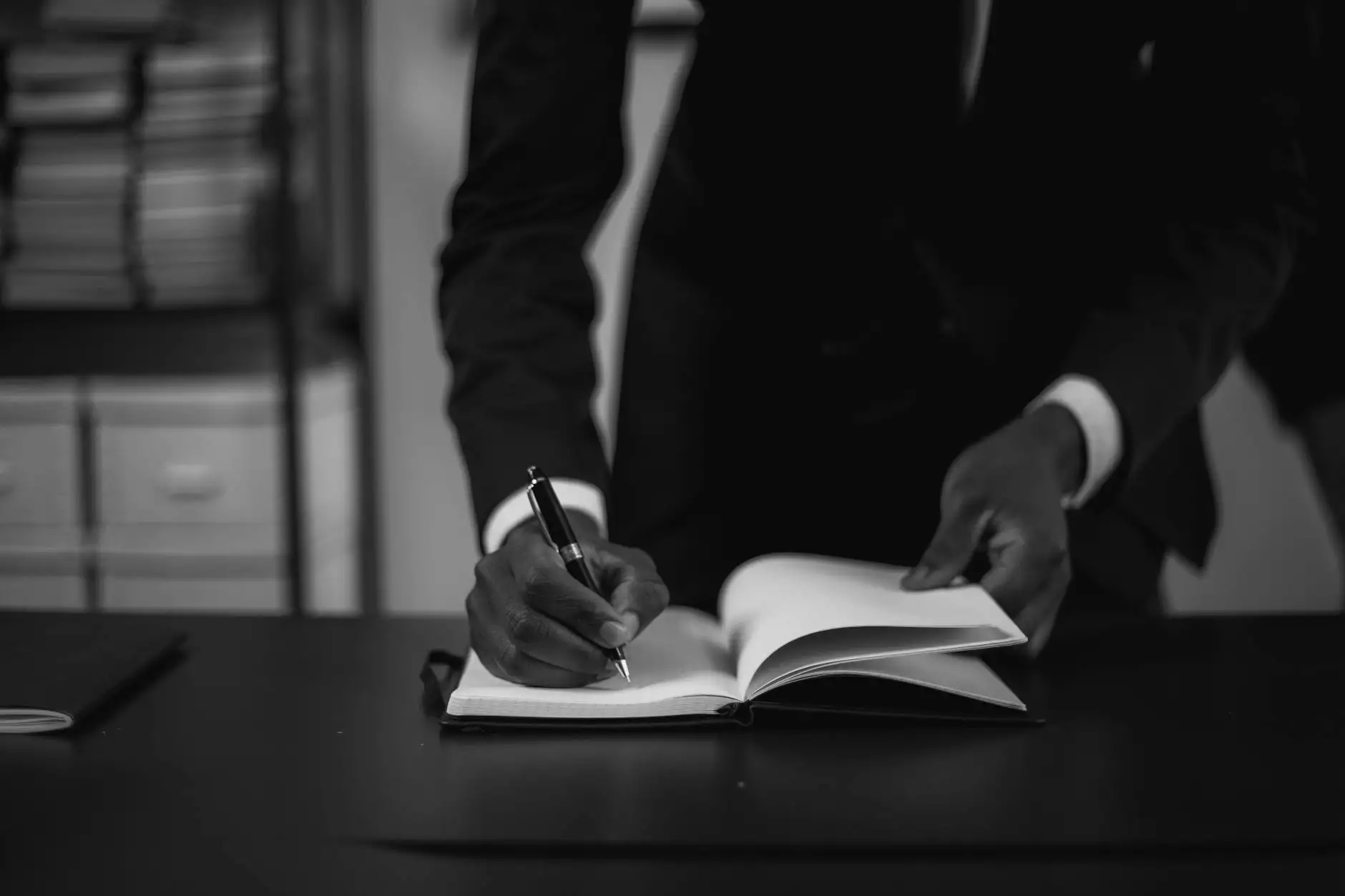
In today's rapidly evolving technological landscape, businesses are increasingly turning to machine learning data annotation as a powerful tool to drive growth and improve efficiency. Whether you operate in Home Services, Keys & Locksmiths, or any other industry, understanding and implementing machine learning can provide a significant competitive edge. This article will delve deep into how machine learning data annotation can transform your business operations, enhance service delivery, and ultimately lead to higher customer satisfaction.
Understanding Machine Learning Data Annotation
Machine learning data annotation involves the process of labeling data to train machine learning models. By accurately labeling data, businesses can develop algorithms that predict outcomes, automate processes, and enhance decision-making capabilities. Proper data annotation is crucial because the effectiveness of a machine learning model heavily relies on the quality and quantity of the labeled data it is trained on.
The Role of Data Annotation in Machine Learning
Data annotation serves as the bridge between raw data and actionable insights. Here are some key aspects related to its role in machine learning:
- Accuracy: Precision in labeling directly impacts the quality of machine learning models.
- Variety of Data Types: Data can be images, text, audio, or video, requiring different annotation techniques.
- Automated vs. Manual Annotation: Depending on the complexity, some data can be annotated manually, while others can be automated using AI tools.
The Importance of Machine Learning in Business
Machine learning technology is being harnessed across various sectors to enhance operational processes, provide insights, and facilitate data-driven decisions. In domains like Home Services and Keys & Locksmiths, machine learning can significantly improve service quality and customer engagement, as discussed below.
1. Optimizing Service Delivery
By implementing machine learning algorithms trained on well-annotated data, businesses in home services and locksmith sectors can optimize their service delivery:
- Predictive Analysis: Predicting customer needs based on previous interactions can lead to more efficient service schedules.
- Personalized Services: Utilizing customer data to offer tailored services can enhance customer loyalty and satisfaction.
2. Enhancing Customer Experience
With improved data annotation processes, companies can leverage machine learning to analyze customer feedback and preferences:
- Sentiment Analysis: Understanding customer sentiment through social media and feedback analysis helps in swiftly addressing concerns.
- 24/7 Customer Support: Automated chatbots trained on conversational data can provide immediate assistance to customers.
3. Streamlining Operations
Machine learning combined with proper data annotation can automate manual tasks, freeing up resources for more critical work. This can include:
- Inventory Management: Predicting stock needs based on customer demand patterns helps in maintaining appropriate inventory levels.
- Route Optimization: For locksmiths, using data to find the most efficient routes can reduce travel time and increase the number of jobs per day.
Implementing Machine Learning Data Annotation in Business
To effectively harness the power of machine learning, businesses must understand how to implement data annotation strategically. Here are steps to consider:
1. Identifying Data Needs
Businesses must first identify what data needs to be annotated. Whether it's service histories, customer inquiries, or market trends, different types of data require different approaches. Careful consideration of what data is valuable will lead to more informed decisions:
- Customer Interaction Data: Analyze past service calls, inquiries, and feedback.
- Geographical Data: Understanding regional service demands can guide geographic marketing efforts.
2. Selecting the Right Annotation Tools
Choosing the right tools for annotation is crucial. There are various tools available, ranging from manual annotation platforms to sophisticated automated solutions:
- Manual Annotation Tools: Useful for precise labeling but can be time-consuming.
- Automated Annotation Software: Leverages AI for faster processing, but initial setup might require training data.
3. Building a Skilled Annotation Team
If opting for manual annotation, businesses must build a team skilled in data analysis and familiar with machine learning concepts:
- Training and Development: Continuous skill development for team members ensures quality annotation practices.
- Quality Assurance: Implementing a review process to maintain high annotation standards is essential.
4. Continuous Improvement and Feedback Loops
Machine learning models improve with continuous feedback and updated data. It's important to establish a system that regularly revisits and refines annotation practices:
- Feedback Mechanisms: Regularly gather feedback from both customers and data annotators to improve processes.
- Iteration: Regularly update your models with new and relevant data for continual learning.
Challenges and Considerations in Machine Learning Data Annotation
While machine learning offers tremendous benefits, businesses must also be aware of potential challenges:
1. Data Privacy and Compliance
When dealing with customer data, businesses must ensure that all data annotation practices comply with regulations such as GDPR.
2. Scaling Annotation Efforts
As businesses grow, the volume of data to annotate can become overwhelming. It’s important to implement scalable solutions early on.
3. Ensuring Data Quality
Without high-quality data, even the most advanced machine learning systems can fail. It’s crucial to prioritize data accuracy at every level.
The Future of Machine Learning Data Annotation in Business
The future of machine learning data annotation is promising. With advancements in AI and machine learning technologies, businesses will continue to optimize their operational efficiencies:
- AI-Powered Annotation: As machine learning algorithms improve, they will increasingly take on data annotation tasks with minimal human intervention.
- Collaborative Annotation Platforms: These will enable businesses to share insights and best practices, thereby driving faster learning cycles.
Conclusion
In conclusion, the implementation of machine learning data annotation is not just a trend; it is a necessity for businesses looking to thrive in a competitive environment. Companies involved in Home Services and Keys & Locksmiths can leverage this technology to improve service delivery, enhance customer experience, and streamline operations. By understanding the principles of data annotation and integrating them into their business strategies, organizations can set themselves up for sustained success.
In summary, investing in machine learning and data annotation is an investment in the future. It opens up new avenues for growth and efficiency that can redefine how businesses operate and succeed in today’s market.